x n m p y
1 1.5 25 8 0.32 -0.753771802
2 2.5 32 13 0.40625 -0.379489622
3 3.5 58 26 0.448275862 -0.207639365
4 4.5 52 22 0.423076923 -0.310154928
5 5.5 43 20 0.465116279 -0.139761942
6 6.5 39 22 0.564102564 0.257829109
7 7.5 28 16 0.571428571 0.287682072
8 8.5 21 12 0.571428571 0.287682072
9 9.5 15 10 0.666666667 0.693147181
其中,x为年家庭收入(万元),n为签订意向书人数,m为实际购房人数,
(1) Logistic回归模型
R实现
————————–
R 实现结果
Call:
lm(formula = logit ~ x)
Residuals:
Min 1Q Median 3Q Max
-0.150322 -0.110376 0.005474 0.117288 0.133341
Coefficients:
Estimate Std. Error t value Pr(>|t|)
(Intercept) -0.88627 0.10242 -8.653 5.50e-05 ***
x 0.15580 0.01686 9.242 3.59e-05 ***
—
Signif. codes: 0 ‘***’ 0.001 ‘**’ 0.01 ‘*’ 0.05 ‘.’ 0.1 ‘ ’ 1
Residual standard error: 0.1306 on 7 degrees of freedom
Multiple R-squared: 0.9243, Adjusted R-squared: 0.9134
F-statistic: 85.42 on 1 and 7 DF, p-value: 3.588e-05
———————-
结果解释:
利用该模型可以对购房概率进行预测。例如当
从而可知年收入8万元的家庭预计实际购房比例为59%。
2.未组数据的Logistic回归
在一次关于在一次关于公共交通的 会调查中,一个调查项目是“是乘坐公共汽车上下班,还是骑自行车上下班”。因变量y=1表示要乘坐公共汽车上下班,y=0表示要乘自行车上下班。自变量x1是年龄,作为连续型变量;x2是月收入;x3是性别,x3=1表示男性,x3=0表示女性。调查对象为工薪族群体,数据如下表。试建立y与自变量间的Logistic回归。
num x3 x1 x2 y num x 3 x1 x3 y
1 0 18 850 0 15 1 20 1000 0
2 0 21 1200 0 16 1 25 1200 0
3 0 23 850 1 17 1 27 1300 0
4 0 23 950 1 18 1 28 1500 0
5 0 28 1200 1 19 1 30 950 1
6 0 31 850 0 20 1 32 1000 0
7 0 36 1500 1 21 1 33 1800 0
8 0 42 1000 1 22 1 33 1000 0
9 0 46 950 1 23 1 38 1200 0
10 0 48 1200 0 24 1 41 1500 0
11 0 55 1800 1 25 1 45 1800 1
12 0 56 2100 1 26 1 48 1000 0
13 0 58 1800 1 27 1 52 1500 1
14 1 18 850 0 28 1 56 1800 1
(1) Logistic 回归模型
因变量
显然
(2)参数
其中
(3)R实现
>datax#数据读入到data3.1中
>glm.logit
#建立y关于x的logistic回归模型,数据为data3.1
>summary(glm.logit)
#模型汇总,给出模型回归系数的估计和显著性检验等
结果显示:
————————
Call:
glm(formula
= y ~ x1 + x2 + x3, family = binomial, data = data3.1)
Deviance
Residuals:
Min 1Q Median 3Q Max
-2.1090 -0.7486 -0.2850 0.7011 2.1683
Coefficients:
Estimate Std. Error z value Pr(>|z|)
(Intercept) -3.655016 2.091218 -1.748 0.0805 .
x1 0.082168 0.052119 1.577 0.1149
x2 0.001517 0.001865 0.813 0.4160
x3 -2.501844 1.157815 -2.161 0.0307 *
Signif.
codes: 0 ‘***’ 0.001 ‘**’ 0.01 ‘*’ 0.05‘.’ 0.1 ‘ ’ 1
(Dispersion parameter for binomial family taken to be 1)
Null deviance: 38.673 on 27 degrees of freedom
Residual
deviance: 25.971 on 24 degrees of freedom
AIC:
33.971
Number
of Fisher Scoring iterations: 5
-------------
结果解释与分析:
输出结果中x2最不显著,决定将其剔除。用y对性别和年龄两个自变量作回归,R程序和输出的结果分别为:
>glm.logit
>summary(glm.logit)
------------
Coefficients:
Estimate Std. Error z value
Pr(>|z|)
(Intercept) -2.6285 1.5537 -1.692 0.0907 .
x1 0.1023 0.0458 2.233 0.0256 *
x3 -2.2239 1.0476 -2.123 0.0338 *
—
Signif.
codes: 0 ‘***’ 0.001 ‘**’ 0.01 ‘*’ 0.05‘.’ 0.1 ‘ ’ 1
------------
结果分析与解释:
可以看出,x3,x1都是显著的。Logistic回归方程为
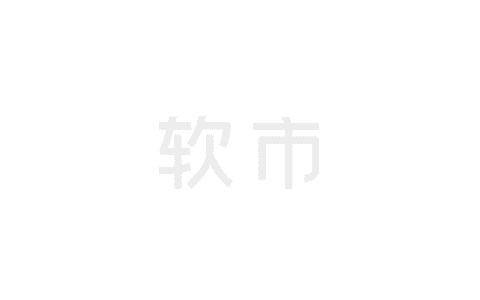
声明:本站部分文章及图片源自用户投稿,如本站任何资料有侵权请您尽早请联系jinwei@zod.com.cn进行处理,非常感谢!