fast and accurate state-of-the-art bivariate kernel density estimator
软件应用简介
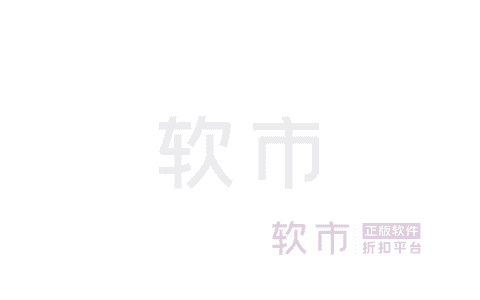
fast and accurate state-of-the-art bivariate kernel density estimator with diagonal bandwidth matrix.
The kernel is assumed to be Gaussian. The two bandwidth parameters are chosen optimally without ever
using/assuming a parametric model for the data or any “rules of thumb”. Unlike many other procedures, this one
is immune to accuracy failures in the estimation of multimodal densities with widely separated modes (see examples).
INPUTS:
data – an N by 2 array with continuous data
n – size of the n by n grid over which the density is computed
n has to be a power of 2, otherwise n=2^ceil(log2(n)); the default value is 2^8;
MIN_XY,MAX_XY – limits of the bounding box over which the density is computed;
the format is:
MIN_XY=[lower_Xlim,lower_Ylim]
MAX_XY=[upper_Xlim,upper_Ylim].
The dafault limits are computed as:
MAX=max(data,[],1); MIN=min(data,[],1); Range=MAX-MIN;
MAX_XY=MAX+Range/4; MIN_XY=MIN-Range/4;
OUTPUT:
bandwidth – a row vector with the two optimal bandwidths for a bivaroate Gaussian kernel;
the format is:
bandwidth=[bandwidth_X, bandwidth_Y];
density – an ‘n’ by ‘n’ matrix containing the density values over the ‘n’ by ‘n’ grid;
density is not computed unless the function is asked for such an output;
X,Y – the meshgrid over which the variable “density” has been computed;
the intended usage is as follows:
surf(X,Y,density)
Example (simple Gaussian mixture)
clear all
% generate a Gaussian mixture with distant modes
data=[randn(500,2);
randn(500,1)+3.5, randn(500,1);];
% call the routine
[bandwidth,density,X,Y]=kde2d(data);
% plot the data and the density estimate
contour3(X,Y,density,50), hold on
plot(data(:,1),data(:,2),’r.’,’MarkerSize’,5)
Example (Gaussian mixture with distant modes):
clear all
% generate a Gaussian mixture with distant modes
data=[randn(100,1), randn(100,1)/4;
randn(100,1)+18, randn(100,1);
randn(100,1)+15, randn(100,1)/2-18;];
% call the routine
[bandwidth,density,X,Y]=kde2d(data);
% plot the data and the density estimate
surf(X,Y,density,’LineStyle’,’none’), view([0,60])
colormap hot, hold on, alpha(.8)
set(gca, ‘color’, ‘blue’);
plot(data(:,1),data(:,2),’w.’,’MarkerSize’,5)
Example (Sinusoidal density):
clear all
X=rand(1000,1); Y=sin(X*10*pi)+randn(size(X))/3; data=[X,Y];
% apply routine
[bandwidth,density,X,Y]=kde2d(data);
% plot the data and the density estimate
surf(X,Y,density,’LineStyle’,’none’), view([0,70])
colormap hot, hold on, alpha(.8)
set(gca, ‘color’, ‘blue’);
plot(data(:,1),data(:,2),’w.’,’MarkerSize’,5)
界面展示
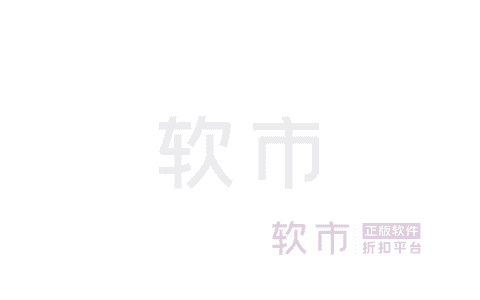
结果示意
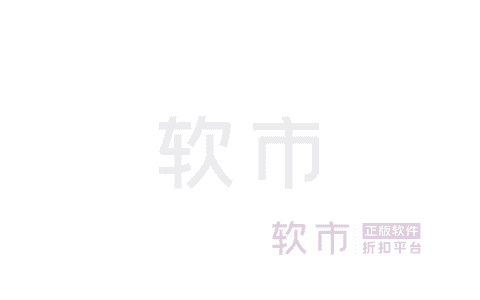
规格 价
0元试用 |
---|
0.0元人民币/月 |
声明:本站部分文章及图片源自用户投稿,如本站任何资料有侵权请您尽早请联系jinwei@zod.com.cn进行处理,非常感谢!